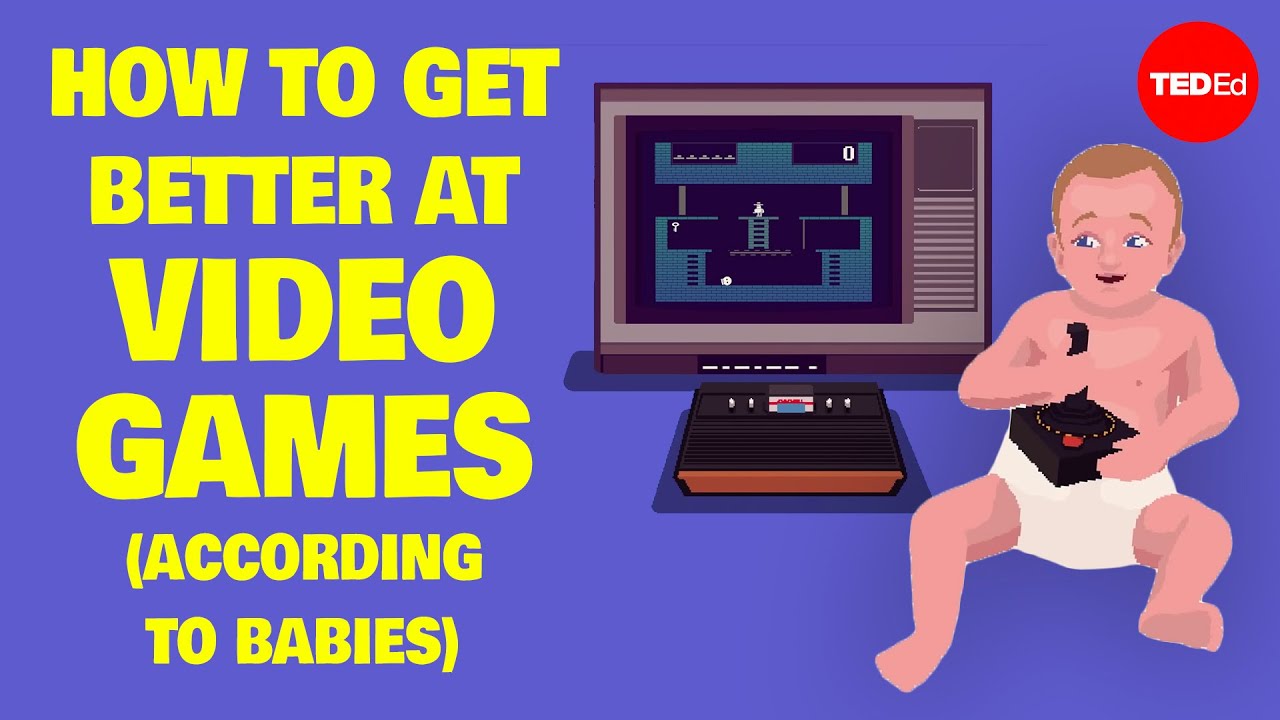
How to get better at video games, according to babies - Brian Christian
In 2013, a group of researchers
at DeepMind in London
had set their sights on a grand challenge.
They wanted to create an AI system
that could beat,
not just a single Atari game,
but every Atari game.
They developed a system they called
Deep Q Networks, or DQN,
and less than two years later,
it was superhuman.
DQN was getting scores 13 times better
than professional human games testers
at “Breakout,”
17 times better at “Boxing,”
and 25 times better at “Video Pinball.”
But there was one notable, and glaring,
exception.
When playing “Montezuma’s Revenge”
DQN couldn’t score a single point,
even after playing for weeks.
What was it that made this particular game
so vexingly difficult for AI?
And what would it take to solve it?
Spoiler alert: babies.
We’ll come back to that in a minute.
Playing Atari games with AI involves
what’s called reinforcement learning,
where the system is designed to maximize
some kind of numerical rewards.
In this case, those rewards were
simply the game's points.
This underlying goal drives the system
to learn which buttons to press
and when to press them
to get the most points.
Some systems use model-based approaches,
where they have a model of the environment
that they can use to predict
what will happen next
once they take a certain action.
DQN, however, is model free.
Instead of explicitly modeling
its environment,
it just learns to predict,
based on the images on screen,
how many future points it can expect
to earn by pressing different buttons.
For instance, “if the ball is here
and I move left, more points,
but if I move right, no more points.”
But learning these connections requires
a lot of trial and error.
The DQN system would start
by mashing buttons randomly,
and then slowly piece together
which buttons to mash when
in order to maximize its score.
But in playing “Montezuma’s Revenge,”
this approach of random button-mashing
fell flat on its face.
A player would have to perform
this entire sequence
just to score their first points
at the very end.
A mistake? Game over.
So how could DQN even know
it was on the right track?
This is where babies come in.
In studies, infants consistently look
longer at pictures
they haven’t seen before
than ones they have.
There just seems to be something
intrinsically rewarding about novelty.
This behavior has been essential
in understanding the infant mind.
It also turned out to be the secret
to beating “Montezuma’s Revenge.”
The DeepMind researchers worked
out an ingenious way
to plug this preference for novelty
into reinforcement learning.
They made it so that unusual or new images
appearing on the screen
were every bit as rewarding
as real in-game points.
Suddenly, DQN was behaving totally
differently from before.
It wanted to explore the room it was in,
to grab the key and escape
through the locked door—
not because it was worth 100 points,
but for the same reason we would:
to see what was on the other side.
With this new drive, DQN not only
managed to grab that first key—
it explored all the way through 15
of the temple’s 24 chambers.
But emphasizing novelty-based rewards
can sometimes create more problems
than it solves.
A novelty-seeking system that’s played
a game too long
will eventually lose motivation.
If it’s seen it all before,
why go anywhere?
Alternately, if it encounters, say,
a television, it will freeze.
The constant novel images
are essentially paralyzing.
The ideas and inspiration here
go in both directions.
AI researchers stuck
on a practical problem,
like how to get DQN to beat
a difficult game,
are turning increasingly to experts
in human intelligence for ideas.
At the same time,
AI is giving us new insights
into the ways we get stuck and unstuck:
into boredom, depression, and addiction,
along with curiosity, creativity,
and play.